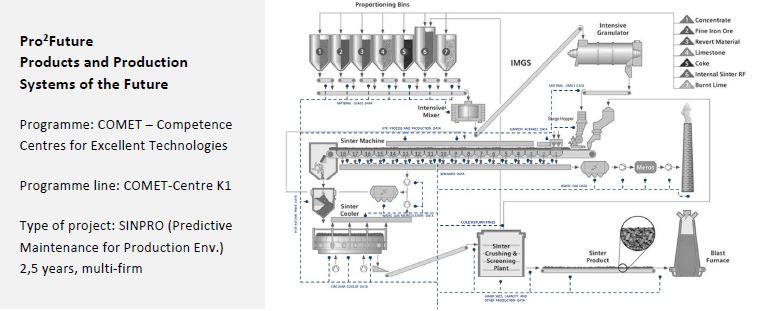
A forecasting Model-Based Discovery of Causal Links of Key Influencing Performance Quality Indicators in Sinter Production
Sintering is a complex production process where the process stability and product quality depend on various parameters. Building a forecasting model improves this process. Artificial Intelligence (AI) approaches show promising results in comparison to current physical models, although often considered as black-box models because of their hidden layers. Due to their complexity and limited traceability, it is difficult to draw conclusions for real sinter processes and improve the physical models in a running plant. This challenge is addressed by focusing on detecting causal links from AI-based forecasting models in order to improve the understanding of sintering and optimizing existing physical models.
In the first step a forecasting model was developed to predict the harmonic diameter as a central quality parameter indicating the grain sizes distribution of the finished sinter. This forecasting model is a ML (machine learning) regression model based on the Random Forest ensemble method. Additionally, approaches such as Support Vector Machine regressor, Multilayer perceptron and K Nearest Neighbours regressor were evaluated. After an optimization step, the model showed a normalized root mean square error of 8.9% (equivalates to 0.2mm) on the prediction of the target value (grain size), which is beyond state of the art.
All Success Stories available via Research > COMET SUCCESS STORIES.